Crowd Activity Change Point Detection in Videos via Graph Stream Mining.
IEEE Computer Society Conference on Computer Vision and Pattern Recognition Workshops(2018)
摘要
In recent years, there has been a growing interest in detecting anomalous behavioral patterns in video. In this work, we address this task by proposing a novel activity change point detection method to identify crowd movement anomalies for video surveillance. In our proposed novel framework, a hyperspherical clustering algorithm is utilized for the automatic identification of interesting regions, then the density of pedestrian flows between every pair of interesting regions over consecutive time intervals is monitored and represented as a sequence of adjacency matrices where the direction and density of flows are captured through a directed graph. Finally, we use graph edit distance as well as a cumulative sum test to detect change points in the graph sequence. We conduct experiments on four real-world video datasets: Dublin, New Orleans, Abbey Road and MCG Datasets. We observe that our proposed approach achieves a high F-measure, i.e., in the range [0.7, 1], for these datasets. The evaluation reveals that our proposed method can successfully detect the change points in all datasets at both global and local levels. Our results also demonstrate the efficiency and effectiveness of our proposed algorithm for change point detection and segmentation tasks.
更多查看译文
关键词
change points,segmentation tasks,crowd activity change point detection,graph stream mining,growing interest,anomalous behavioral patterns,novel activity change,crowd movement anomalies,video surveillance,hyperspherical clustering algorithm,consecutive time intervals,directed graph,graph edit distance,cumulative sum test,graph sequence,real-world video datasets,MCG Datasets,Dublin dataset,New Orleans dataset,Abbey Road dataset,MCG dataset
AI 理解论文
溯源树
样例
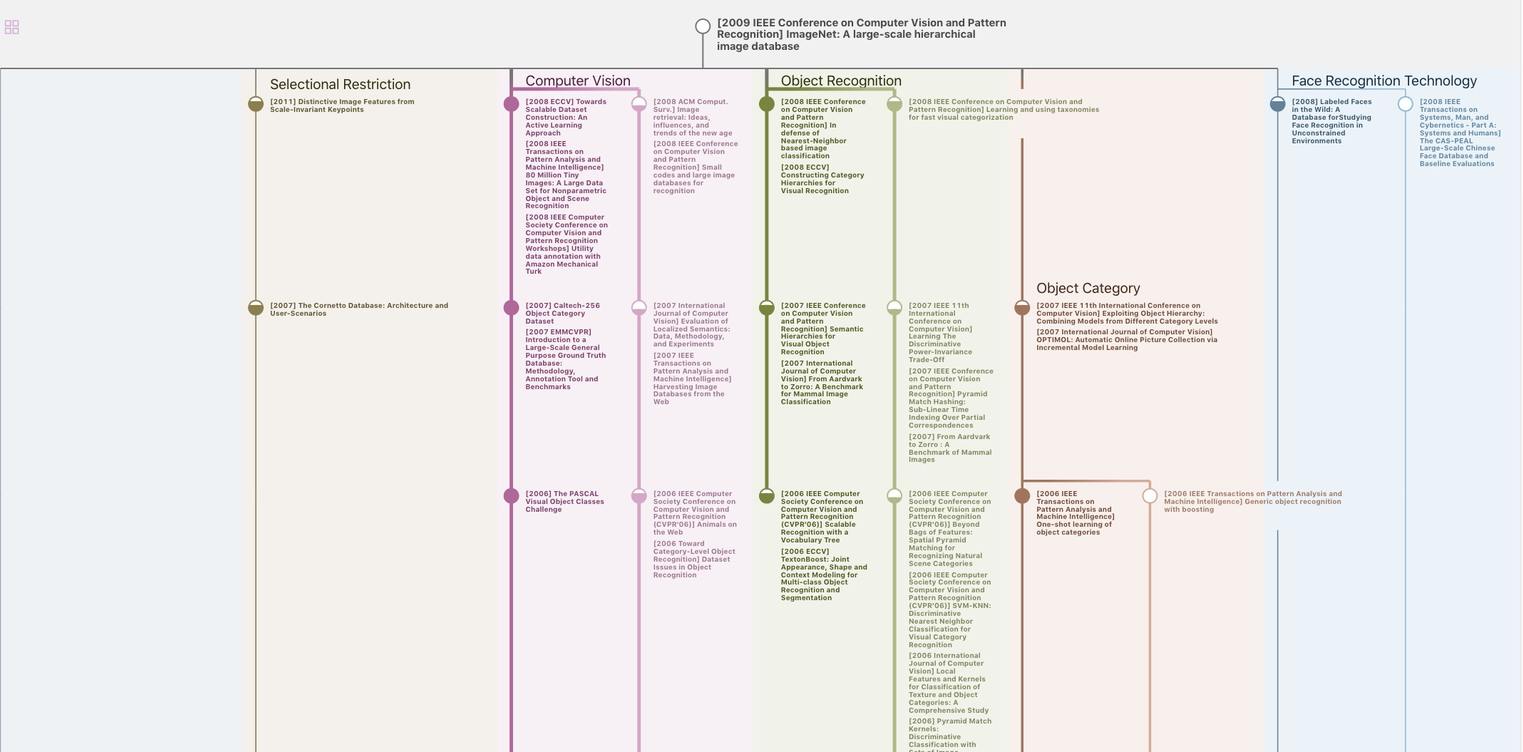
生成溯源树,研究论文发展脉络
Chat Paper
正在生成论文摘要