A Survey on Anomaly detection in Evolving Data
Sigkdd Explorations(2018)
摘要
Traditionally most of the anomaly detection algorithms have been designed for 'static' datasets, in which all the observations are available at one time. In non-stationary environments on the other hand, the same algorithms cannot be applied as the underlying data distributions change constantly and the same models are not valid. Hence, we need to devise adaptive models that take into account the dynamically changing characteristics of environments and detect anomalies in 'evolving' data. Over the last two decades, many algorithms have been proposed to detect anomalies in evolving data. Some of them consider scenarios where a sequence of objects (called data streams) with one or multiple features evolves over time. Whereas the others concentrate on more complex scenarios, where streaming objects with one or multiple features have causal/non-causal relationships with each other. The latter can be represented as evolving graphs. In this paper, we categorize existing strategies for detecting anomalies in both scenarios including the state-of-the-art techniques. Since label information is mostly unavailable in real-world applications when data evolves, we review the unsupervised approaches in this paper. We then present an interesting application example, i.e., forest re risk prediction, and conclude the paper with future research directions in this eld for researchers and industry.
更多查看译文
AI 理解论文
溯源树
样例
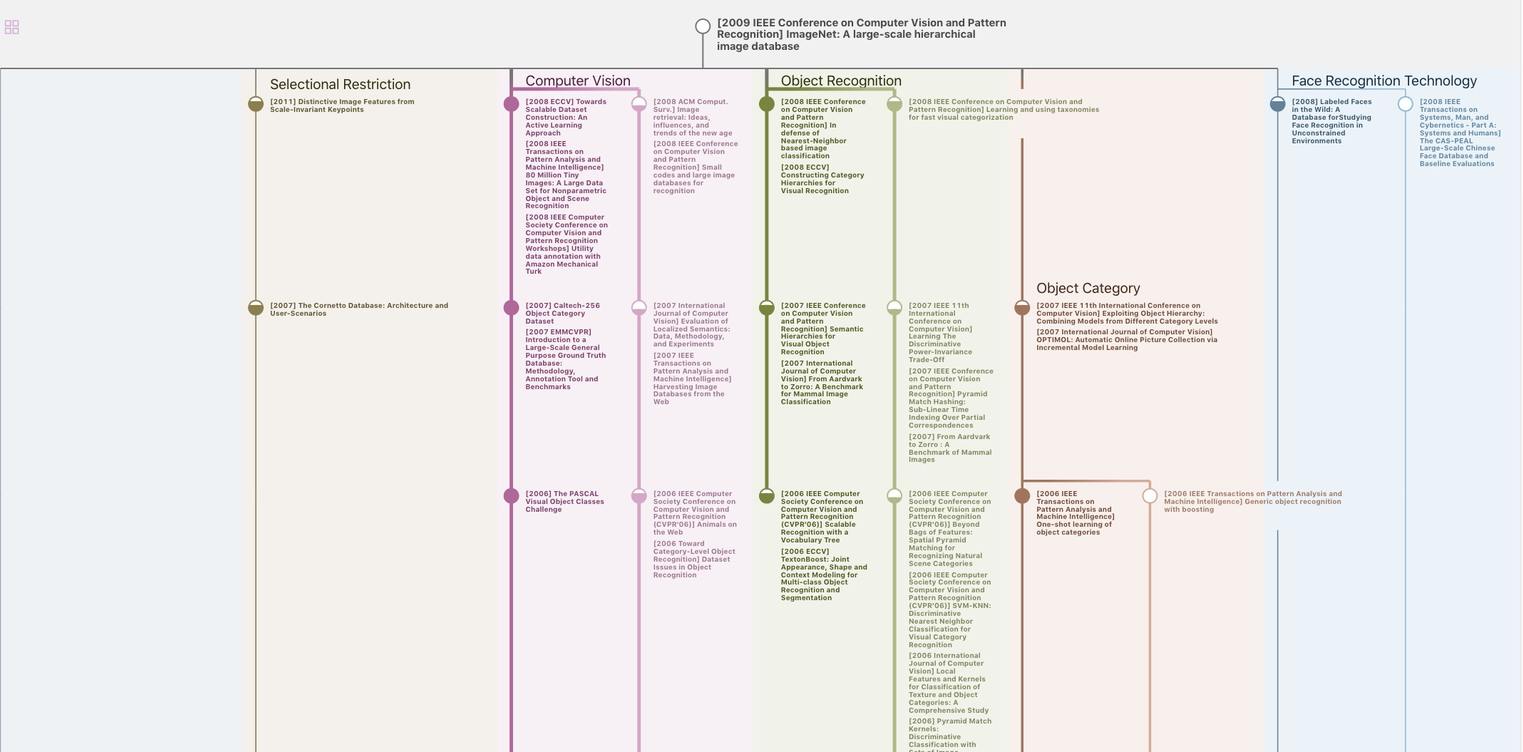
生成溯源树,研究论文发展脉络
Chat Paper
正在生成论文摘要