When Measurement Misleads: The Limits of Batch Assessment of Retrieval Systems
SIGIR Forum(2023)
摘要
The discipline of information retrieval (IR) has a long history of examination of how best to measure performance. In particular, there is an extensive literature on the practice of assessing retrieval systems using batch experiments based on collections and relevance judgements. However, this literature has only rarely considered an underlying principle: that measured scores are inherently incomplete as a representation of human activity, that is, there is an innate gap between measured scores and the desired goal of human satisfaction. There are separate challenges such as poor experimental practices or the shortcomings of specific measures, but the issue considered here is more fundamental - straightforwardly, in batch experiments the human-machine gap cannot be closed. In other disciplines, the issue of the gap is well recognised and has been the subject of observations that provide valuable perspectives on the behaviour and effects of measures and the ways in which they can lead to unintended consequences, notably Goodhart's law and the Lucas critique. Here I describe these observations and argue that there is evidence that they apply to IR, thus showing that blind pursuit of performance gains based on optimisation of scores, and analysis based solely on aggregated measurements, can lead to misleading and unreliable outcomes.
更多查看译文
AI 理解论文
溯源树
样例
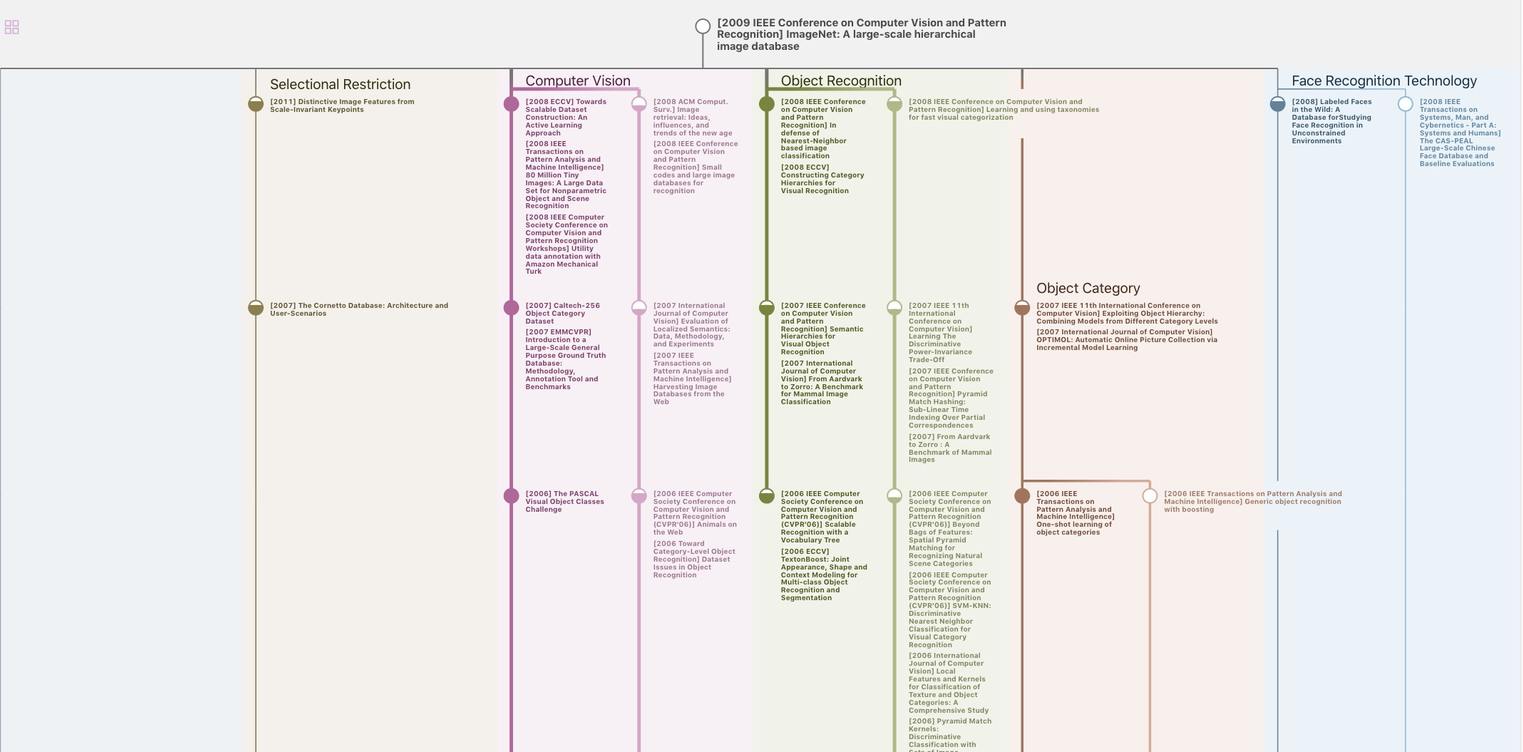
生成溯源树,研究论文发展脉络
Chat Paper
正在生成论文摘要